Enhancing Sydney Water’s leak prevention through acoustic monitoring
By R Nikoloska, L Bykerk, D Vitanage, J V Miro, F Chen, Y Wang, B Liang and S Verma.
First published in Water e-Journal Vol 5 No 2 2020.
Abstract
Leaks and breaks pose a great challenge for every water network around the world. These challenges include disruptions to customer services, costly pipe maintenance and unaccounted water loss. With ever-advancing smart technology and the rise of the Internet of Things (IoT), acoustic sensors, as a method of leak detection, can effectively identify potential leaks for proactive and targeted repairs.
As part of its Active Leak Detection (ALD) program, Sydney Water in collaboration with the University of Technology Sydney (UTS) have deployed 204 acoustic sensors since December 2019 across five Central Business District (CBD) areas. The Sydney CBD, Bankstown, Penrith, Chatswood and Liverpool have been prioritised for sensor deployment through the Sydney Water-UTS pipe failure prediction tool model.
Since deployment, 35 leaks have been detected, of which 21 have been confirmed (inclusive of a main break) and repaired. 70% of these leaks have matched with the pipe failure prediction tool model.
During this period, Sydney Water has also been collaborating with UTS to progress development of advanced acoustic analysis to improve the interpretation of data and confidence in results. This analysis will be translated into an automated machine learning algorithm and a leak detection portal to host the range of acoustic sensor models in the one location for ease and efficiency of integration within Sydney Water.
This paper discusses the selection of zones for sensor deployment, the deployment of different models of acoustic sensors within Sydney Water’s system, and the change to Sydney Water’s business-as-usual network operations to ensure leak detection success. It also highlights the need for integrated advanced analytics to increase the confidence in identifying potential leaks and breaks.
Introduction
With around 22,000 km of water pipes in Sydney Water’s network, Sydney Water experiences around 6,500 annual leaks and breaks resulting in an 8% unaccounted water loss. Of these, 70% are leaks in small distribution pipes and 175 of those breaks are on critical water mains, with the total renewal costs amounting to $80 million, including maintenance.
As part of the Sydney Water’s Active Leak Detection (ALD) program, there is an ongoing need to reduce disruptions to customers, identify unaccounted water loss from the supply network, reduce costs of repair maintenance and to ensure water is reserved for customer consumption to support ongoing drought resilience efforts within NSW.
What is understood from water networks around the world is that overall leakage from hidden leaks and undetected breaks is significantly greater than from those that are reported breaks (Samir, Kansoh, Elbarki and Fleifle, 2017).
The benefits of detecting leaks within the Sydney Water system is thus two-fold; 1) detecting leaks before a main break to reduce disruption to the network and customers, and 2) detecting hidden leaks which never surface to reduce unaccounted water loss.
The last decade has seen a rise in the use of the Internet of Things (IoT) to support city management with the development of so-called smart cities (Rojek and Studzinski, 2019). To achieve successful leak detection by similar means, one method currently under development is acoustic monitoring capabilities. Acoustic sensors are constantly improving and provide a viable solution to remotely detect hidden leaks before a main break.
Since December 2019, Sydney Water, in collaboration with the University of Technology Sydney (UTS) has deployed 204 acoustic sensors across five CBD areas. These locations have been prioritised by UTS’ pipe failure prioritisation tool.
Current process
Currently, Sydney Water relies heavily on its customers and employees to report surface water from a leak or break. This reactive approach to fixing leaks and breaks needs to be improved in order to enhance Sydney Water’s customer experience.
With ageing infrastructure and a growing population, Sydney Water’s network will continue to be susceptible to leaks and breaks. As water pipelines are located deep underground, until water surfaces or a leak turns into a main break, leaks remain hidden and are unable to be repaired (Liu et al., 2019). These leaks are known as background leaks, with some leaks never surfacing and instead continuing to leak within the pipe, causing unaccounted water loss (Hamilton and Charalambous, 2020).
Sydney Water’s ALD program aims to maintain leakage below the Economic Level of Leakage (ELL) target of 105 ml/day. As part of the ALD program, surveying of assets entails specialist leak surveyors inspecting water reticulation visually and testing for the characteristic noise of leaks using acoustic equipment such as listening sticks (El-Zahab and Zayed, 2019). This method relies on the ability of the operations team to listen and use their judgement to pinpoint a leak.
Depending on the material type of the pipes, listening sticks can be effective, however the accuracy and efficiency of determining a leak sound is influenced by external noise and relies heavily on human sense and experience (Hamilton and Charalambous, 2020).
Replacing this method with advancing leak detection sensor technologies allows for Sydney Water’s network operations team to place all their efforts on repairing the pipe in a proactive and efficient manner.
Pipe failure prioritisation tool
A pipe failure prioritisation prediction tool based on the assessment of Minimum Night Flow (MNF) has been developed to integrate multi-source data collected from Sydney Water and public data sources to assist with sensor deployment.
MNF for a pressure zone is defined as the water flow measured between 1 am to 5 am, connected to industry, commercial and residential demand. The motivation for choosing this time range is that the amount of water consumption is minimal over the whole day. This model enhances the “situational awareness” of network and operations.
Further data analysis has been conducted using the developed tool at a pressure zone level and pipe level. The model of the tool is trained using the latest Sydney Water historical records. The trained model can produce failure likelihoods for various levels: pipes, shutdown blocks, and trunk mains. Hence, the prediction results from the model have been leveraged to prioritise zones and pipes to assist sensor deployment.
The failure prediction tool has been evaluated using all pipes and large pipes based on historical records alone. The evaluation results are shown in Figure 1, showing the performance on all pipes. The x-axis represents the cumulative percentage of the inspected water pipes, and the y-axis indicates the percentage of the detected pipe failures. If 20% of pipes of the priority list are inspected, about 80% of failures can be detected.
To prioritise zones/areas for sensor deployment, a priority map at zone level is also obtained based on the failure likelihoods from the pipe failure prediction tool. Specifically, a ranking score of the pressure zone is aggregated by summing up all failure likelihoods of the pipes in that zone.
As illustrated in Figure 2, all pressure zones are colour coded to represent different priority levels. In addition, the top 10 CBDs are listed. Four highlighted CBDs (Sydney, Liverpool, Bankstown, and Penrith) have been selected for acoustic sensor deployment.
In addition to the priority map at zone level, it is essential to generate priority maps at pipe level for each zone to guide sensor deployment. Based on the failure likelihood of pipes, the top 5% prioritised pipes are highlighted and coded in different colours. Figure 3 shows an example of a priority map for the Sydney CBD.
Acoustic sensor types and models
Many sensing options exist for leak detection. Static acoustic sensors are one acoustic monitoring technology that can be used as a permanent or semi-permanent solution for leak detection (akin to listening sticks widely used by water utilities). These sensors are fixed onto a pipeline surface and used to sense real information about pipeline resources (Wong et al., 2018).
Many types of static acoustic sensor models exist, each with unique sensing technologies, processes, and suppliers. To gain a comprehensive understanding of how different static acoustic sensors work, four different models have been deployed within Sydney’s five CBD’s as shown in Figure 4.
Each static acoustic sensor model has different data recording capabilities; however, noise recordings are typically made between 2 and 4am, at a time of lower water usage. By recording the audio file at this time every day, environmental noise from water usage and traffic are reduced (but not eliminated), allowing for audio files across multiple days to be compared and monitored for changes. Examples of the different data collection methods for the four different acoustic sensor types are noted in the following subsections.
Detection Services – Von Roll Ortomat loggers: The loggers record a noise level reading (irrespective of frequency band) every day in 5-minute intervals, along with a 10-second audio file at 2:05am every day. All data is available in the online portal ‘HydroPort’ for further analysis. An example of a Von Roll static acoustic sensor is shown in Figure 5.
Detection Services – HWM PermaNET+ loggers: Every day at 3:45am, the loggers record a 10-second audio file. Histograms are generated in the HWM Online portal, noting the leak spread and noise level.
WaterGroup – SebaKMT Log N-3 loggers: Every day between 2 and 4am, the loggers take 100 measurements of the noise level (measured in dB). The loggers also record a 2-second audio file at 2am. The logger will only send the audio file if the lowest measured noise level exceeds a set threshold value. The audio files and histogram data of the 100 measurements can be accessed on the Seba Cloud portal.
Ovarro – Primayer Enigma3m loggers: Every day at 2am, the loggers record a 10-second audio file. The Prime Web portal uses these audio files to analyse and present automatic correlations between the loggers.
All these acoustic sensors will work to gather data utilising their own methods and processes, which will enhance the data received. UTS and Sydney Water have access to all the mentioned portals, and with training from acoustic sensor providers have understood how to interpret the data.
Method for detection and localisation of leaks
To date, a total of 35 leaks have been detected of which 21 have been verified (inclusive of a main break) and repaired. When assessing the location of the verified leaks, it was found 70% overlapped with priority pipes assessed by the Sydney Water-UTS pipe failure prediction model. Table 1 presents a summary of the detected and verified leaks on a per-zone basis.
The automatic leak flags raised from each respective sensor portals were assessed, and the data further analysed before investigations were conducted on-site for leak localisation and repair. Some examples of the online portal leak flags are listed below.
SebaCloud leak example – Liverpool
The histogram shown in Figure 6 from the SebaCloud portal was assessed, where it was found that the logger highlighted in the bottom row was showing a high probability of a leak.
In the histogram shown in SebaCloud, the highlighted logger is shown in a leak state (red) prior to repair taking place. After this, the logger status turns green, indicating low noise levels.
Further analysis by UTS of the available audio files revealed elevated noise levels since the logger was first deployed as shown in Figure 7.
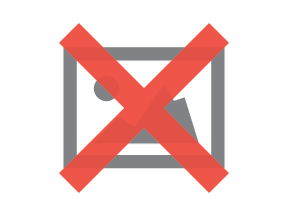
Von Roll HydroPort leak example – Bankstown
Elevated noise levels were being recorded by a Von Roll logger in Bankstown since deployment in mid-March. With an approximate location provided by online correlation of multiple loggers in the HydroPort portal, Sydney Water crews performed on-site correlations to pinpoint and repair the leak.
Figure 8 shows the elevated noise levels which were recorded by the Von Roll logger and shown in HydroPort, prior to the repair taking place on 15 May.
There was a significant reduction in noise after the leak was repaired. The red bars show that the logger was in a leak state prior to repair, with the bars turning green after the repair took place, indicating the logger is not picking up a leak.
HWM online leak example – Penrith
Elevated noise levels were being recorded by an HWM PermaNET+ logger in Penrith since deployment in mid-March, as shown by the leak state of the logger in the HWM Online portal in Figure 9.
With an approximate location provided by online correlation of two loggers in the HWM Online portal (see Figure 10), Sydney Water crews performed on-site correlations to pinpoint and repair the leak.
Significant changes in the audio spectrums at this location have been recorded since the repair was conducted on 27 May.
This is shown in Figure 11 by the spectral analysis of the individual audio files conducted by UTS.
Prior to 27 May, leak noise was evident as a relatively high-power persistent noise in the frequency band around 300 Hz, with other persistent bands around 700, 800 and 1000 Hz.
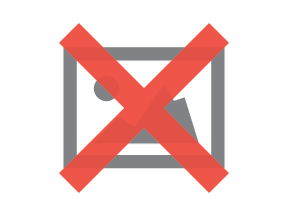
A new, proactive approach to working
Sydney Water developed a process of action to follow up on leaks detected by acoustic sensors by communicating with Sydney Water’s Network Operations team. The team interpret UTS recommendations and reviews of the acoustic sensor data and issue work orders for Sydney Water area network technicians to investigate and repair the leak.
Both UTS and Sydney Water teams are currently working to orient, train and upskill the required teams who are already experiencing changes to the way they work.
Repairing pipes with acoustic sensors is proactive, and this new way of working will optimise the efficiency of area network technicians with work being planned and scheduled. The ability for acoustic sensors to provide an approximate location of the leak also reduces time spent on manual surveying of the water pipe and significantly improves safety.
The two leaks mentioned below were confirmed and successfully repaired through proactive measures of the Sydney Water Network Operations team and the Area Network Technician team. These leaks were repaired at night as external noise is low, allowing for listening sticks and mobile correlators to be used by the network technicians to confirm and pinpoint the location of the leak.
Leak #1 – Dalley Street, Sydney CBD
SA Water assisted with the deployment and analysis of 40 Von Roll acoustic sensors deployed in the Sydney CBD. A leak detected on Dalley Street was first reported on 15 January. Noise produced was loud with the acoustic sensors identifying a leak every few meters in Underwood Street.
Sydney Water crew responded to the leak on 2 March, where the main was isolated prior to excavation. A faulty main tap was found (which was causing a leak of about 3 L/s), removed, and a bolted clamp was installed, as shown in Figure 12.
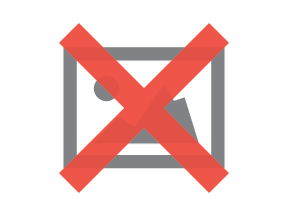
Leak #2 – Speed Street, Liverpool
UTS analysis confirmed a noisy logger with a high probability of a leak. With the acoustic sensor providing an approximate leak location, Sydney Water crews discovered water from the leak was flowing into a nearby stormwater pit at an estimated rate of 2-3 L/s on 19 May.
On 20 May, the leak was pinpointed on-site through correlation, with a hole found in a 50mm copper service under two buried fire service valves as shown in Figure 13. The following day Sydney Water repaired the leak overnight.
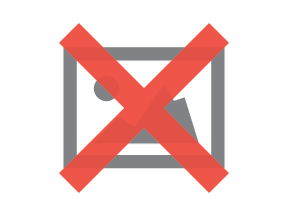
Reducing false positive rates with machine learning
Many water utilities have trialled the use of leak detection systems with much success, with case studies explored in Leak Detection: Technology and Implementation (Hamilton and Charalambous, 2020). Each utility has faced unique challenges based on the material, age, and location of their assets.
Depending on the location of sensor deployment, the magnitude of environmental noise can significantly differ. For example, in Australia, Adelaide’s CBD is vastly different to that of Sydney’s CBD, which can be
attributed to:
- a higher population and an increase in both pedestrian and vehicular traffic,
- a more expansive public transport system – with an underground railway, and the newly commissioned above-ground light rail, and
- a higher number of commercial businesses.
Basic analysis of acoustic sensing data is therefore unreliable in detecting a leak and raising a true positive leak alert. A common approach to reduce the number of false positive leak alerts is to train a machine learning classifier to distinguish a true leak noise from other environmental or water usage noise (El-Zahab et al., 2017, Kang et. Al. 2018., Liu et. Al. 2019).
SA Water is an example of a water utility within Australia which has successfully developed an algorithm to understand leak sounds (Stephens et al., 2019). Without training, vibrations from environmental sources are indistinguishable from the sound of a leak due to their similar frequency spectrum (Liu, Ma, Li, Tie et al., 2019). It is these “non-leak” noises that the system confuses for a leak and causes false positive rates (Hamilton and Charalambous, 2020).
Using the data obtained from the large array of sensors deployed across Sydney, an opportunity has arisen to train and test a machine learning classifier for five different CBD locations. The trained classifier will ideally be able to interpret a variety of environmental noises and accurately determine a leak noise in multiple deployment zones unique to Sydney. As the sensors will remain in-situ, the data collection and verification of leaks from Sydney Water operational teams will be ongoing, allowing for the model to be re-trained and improved as the sensor database grows.
Since each acoustic sensor deployed in the five CBD zones have slightly varying capabilities and available data, UTS is currently developing a method for the consolidated analysis of all data from the various acoustic sensors.
Furthermore, since many of the deployments are in their early stages, the environmental noises recorded by the acoustic sensors are still being analysed and understood. For example, one logger in Bankstown is located near two major stormwater assets, and therefore records significant increases in noise during periods of rainfall.
Currently, the acoustic data is being monitored and possible leaks are being flagged weekly for further investigation by Sydney Water.
Leak detection web portal
To improve the current process of accessing and analysing acoustic sensor data from multiple portals, an integrated sensing and analytics toolkit prototype is to be developed. The aim of this portal is to consolidate the sensing data from various sensor providers through web/cloud-based applications and incorporate multiple data sources from Sydney Water. Having all information under one platform will also enable for this data to be easily transferred into a machine learning system.
The platform is to be Geographic Information System (GIS) based and compatible with Sydney Water’s Information Technology (IT) requirements to produce regular reports on leaks and high-risk assets for the operational teams. The web portal will communicate with sensor providers to set up access to data portals, enabling further data analytics and prediction. The system diagram of the web portal is shown in Figure 14.
Value and benefits
Following the deployment of acoustic sensors, Sydney Water aims to reduce reactive leak detection work by 50% and reduce 50% of unaccounted water loss within three years in the five CBD areas.
These findings will also consolidate the current learnings of UTS and Sydney Water in its predictive MNF and predictive modelling of pipes for leaks to improve its ALD program by reducing 25% of unaccounted water loss in 50% of the pressure zones over three years.
The lack of disruption to Sydney Water’s network will benefit all customers, motorists, residents, and the public with reducing their need to call Sydney Water to report leaks or breaks and reduced disruption to their everyday lives.
It will also reduce the need for manual surveying and increase safety standards for Sydney Water employees as they can better plan and schedule work.
Conclusion
To create a better life with world-class water services, Sydney Water is continually exploring and implementing innovative technologies to improve services to over five million customers across Greater Sydney.
To achieve this, reducing leaks and breaks in Sydney Water’s system by using acoustic sensors will reduce unaccounted water loss, reduce disruptions to its network and increase customer satisfaction. This is a part of an overall smart network asset strategy that has been implemented by Sydney Water.
Next steps involve continuing to interpret the data from the acoustic sensors to train a machine learning model to improve detection of leaks before they turn into breaks. Once an algorithm has been sufficiently trained and validated, a proactive process for the repair and maintenance of leaks and breaks will be integrated into Sydney Water’s business-as-usual practice.
The outcomes of this research study will mean Sydney Water will have a strategy to target acoustic sensing to be more agile and mobile in urban areas, ensuring water distribution that provides 24 hours of reliable and resilient services to our customers.
Acknowledgements
We would like to acknowledge the support and operational intellect of the Sydney Water Network Operations team led by Georgiana Anderson, Abdul Rehman, David Cantlon, Brendan Cantlon, Greg Alford, Andrew Curtis, Pradeep Perera and Chau Nguyen who proactively demonstrated the success of sensing-based leak prevention.
Thank you to Customer Hub representative Nigar Afroza and Asset Life Cycle representatives Johnny Xiao and Aravinda Stanley. We would also like to thank our acoustic sensor suppliers Detection Services, Ovarro and WaterGroup for their continual support.
We also acknowledge the effort undertaken by Mark Stephens and Nicole Arbon from SA Water, including Adrian Cavallaro for installation and NSW Smart Sensing Network (NSSN).
About the authors
Rebeka Nikoloska | Science, Research, and Innovation Project Manager at Sydney Water. Rebeka is using her background in Media and Communications and Marketing from The University of Sydney to coordinate and manage the multi-disciplinary project team. Rebeka coordinates the review of acoustic sensor data within Sydney Water’s network, alongside other large collaborative asset innovation projects related to robotic sensing.
Lili Bykerk | Research Engineer at the University of Technology Sydney (UTS). Lili received her B. Eng degree in mechanical and mechatronic engineering, and her Ph.D. degree in engineering (robotics) from UTS in 2014 and 2019 respectively. Her areas of interest include robotics, sensors and machine learning. Lili is currently working in close collaboration with sensor suppliers and Sydney Water for advanced leak detection with acoustic sensors.
Dammika Vitanage | Asset Infrastructure Research Coordinator for Sydney Water. Dammika has 40 years of water industry experience. Recently Dammika has been the industry lead for large collaborative asset innovation projects. He has extensive experience in water industry operations, planning, research and innovation. Dammika has led significant large research and innovation collaborations and won many awards.
Jaime Valls Miro | Associate Professor at the University of Technology Sydney (UTS). Jaime received his B.Eng. and M.Eng. in Computer Science (Systems Engineering) in 1990 and 1993 respectively. He received his Ph.D. in robotics and control systems in 1998. His areas of interest include robotic sensor modelling for perception and mapping, computational intelligence in HRI and robot navigation. In the last few years, he has devoted this interest in pursuing a better understating of condition assessment sensing for water mains in close collaboration with the water industry.
Fang Chen | Distinguished Professor at University of Technology Sydney. She has created many world-class AI innovations while working in Intel, Motorola, NICTA, CSIRO and UTS. She won the 2016 NSW Water Professional of the Year, and the National and NSW Research and Innovation Award by the Australian Water Association. She received the Brian Shackle Award in 2017 for “the most outstanding contribution with international impact in the field of human interaction with computers and information technology”. She has over 280 publications and 30 patents.
Yang Wang | Associate Professor at University of Technology Sydney as well as a visiting principal researcher of Data61, CSIRO. He received his PhD degree in Computer Science from the National University of Singapore in 2004. Before joining Data61 (formerly NICTA) in 2006, he was with the Institute for Infocomm Research, Rensselaer Polytechnic Institute, and Nanyang Technological University. His research interests include machine learning and information fusion techniques, and their applications to asset management, intelligent infrastructure, cognitive and emotive computing, medical imaging, and computer vision.
Bin Liang | Lecturer at Data Science, University of Technology (UTS). Bin received his PhD degree from Charles Sturt University, Australia in 2016. Before joining UTS, he was a postdoctoral fellow in Data61-CSIRO. He is an Artificial Intelligence developer, researcher, and practitioner, with years of experience in using predictive modelling, data processing, and data mining algorithms to solve business problems. He has conducted research and produced papers at top-tier conferences and journals. His research interests include data mining, computer vision, pattern recognition, machine learning and survival analysis.
Sunny Verma | Postdoctoral Research Fellow at the Data Science Institute, University of Technology Sydney, and as a Visiting Scientist at Data61, CSIRO. He received his Ph.D. degree in Computer Science from University of Technology Sydney in 2020. Before joining UTS, he was a Research Assistant at Department of Electrical Engineering, IITD India, and then worked as Senior Research Assistant at Hong Kong Baptist University, Hong Kong. His research interests include data mining, fairness in machine learning, interpretable deep learning, and open-set recognition systems.
References
El-Zahab, S., Asaad, A., Mohammed Abdelkader, E., Zayed. T, “Collective thinking approach for improving leak detection systems,” Smart Water, vol. 2, no. 3, 2016.
El-Zahab, S., Zayed, T. “Leak detection in water distribution networks: an introductory overview,” Smart Water, vol. 4, no. 5, 2019.
Hamilton, S., Charalambous, B., “Leak detection: Technology and Implementation,” IWA Publishing, London, 2013.
Kang, J. Y. Park, Y., Lee, J., Wang, S. & Eom, D., "Novel Leakage Detection by Ensemble CNN-SVM and Graph-Based Localization in Water Distribution Systems," IEEE Transactions on Industrial Electronics, vol. 65, no. 5, pp. 4279-4289, 2018.
Liu, Y., Ma, X., Li, Y., Tie, Y., Zhang, Y., & Gao, J. “Water Pipeline Leakage Detection Based on Machine Learning and Wireless Sensor Networks,” Sensors (Basel), vol. 19, no. 23, 2019.
Rojek, I. & Studzinksi, J., “Detection and Localization of Water Leaks in Water Nets Supported by an ICT System with Artificial Intelligence Methods as a Way Forward for Smart Cities”, Sustainability, vol. 11, no. 2, 2019.
Samir, N., Kanosh, R., Elkbari, W., & Fleifle, A. “Pressure Control for minimizing leakage in water distribution systems,” Alexandria Engineering Journal, vol. 56, no. 4, 2017.
Wong, L., Deo, R., Rathnayaka, S., Shannon, B., Zhang, C., Kodikara, J., Chiu, W., & Widyastuti, H. “Leak detection and quantification of leak size along water pipe using optical fibre sensors package,” Special Issue: Electronic Journal of Structural Engineering, vol. 18, no. 1, 2018.