Predictive maintenance for a water reuse utility
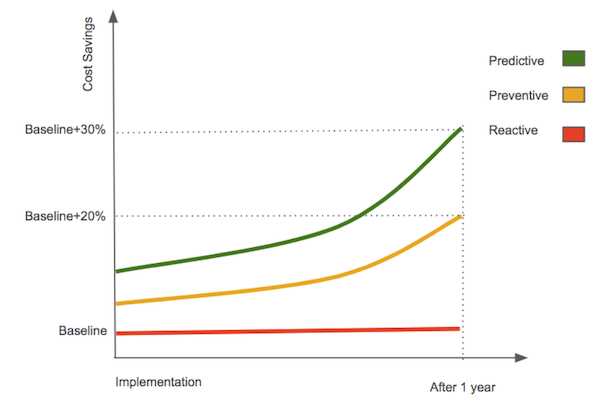
Data is becoming increasingly important for water utilities and councils in terms of allowing for more in-depth insight into asset performance and, in turn, opportunities for more predictive maintenance and investment decisions.
SpiralData recently partnered with the City of Playford in Adelaide’s north to assist in predictive maintenance of pumps using machine learning.
City of Playford’s Playford Water supplies recycled water for irrigation across 70 sites, distributed through 70 km of pipe via a combination of over 30 bore, distribution and jacking pumps.
The managed aquifer recharge schemes include four wetland sites that collect and treat stormwater, which is stored in aquifers and purchased by customers for irrigation purposes during summer months.
City of Playford were looking for a way to transition from a reactive maintenance, refurbishment and replacement strategy, which impacts scheduling and budgeting, towards a predictive maintenance approach.
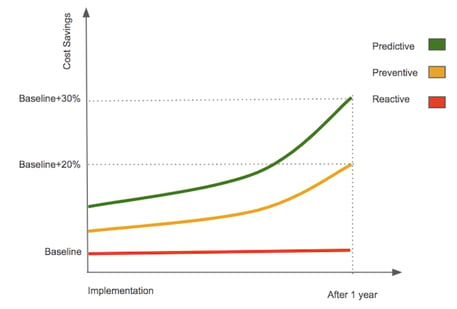
Predictive maintenance generally leads to 30-40% cost savings compared to reactive maintenance, and 10-20% cost savings compared to preventive maintenance.
While preventative maintenance focuses on routine tasks based on expected asset lifecycle, preventative maintenance involves condition monitoring to predict failures, allowing for targeted maintenance when and where it is needed.
While the City of Playford has mature monitoring capabilities via its SCADA system, SpiralData and SAGE Automation built on previous collaborations to assist the council in working towards scheduling efficiency and cost-savings via a predictive approach to pump maintenance for its irrigation networks.
SpiralData leveraged the City of Playford’s dataset – which includes data recorded at 5-minute intervals – utilising its IoT and machine learning reference architecture.
The approach has reduced the time-to-insight for a range of SpiralData customers pursuing solutions suited to machine learning, with the in-built machine learning workbench allowing for rapid prototyping of different algorithms.
Results can be visualised in dashboards, and includes automated alerts sent via SMS and other communication tools – Microsoft Teams or Slack, for example – delivering an end-to-end solution from the device to the decision-maker.
Key insights
Applying SpiralData’s IoT and machine learning architecture allows for three key benefits for the the City of Playford, including automated anomaly detection for turbidity in real-time, identification of the wear profile of each pump, and the potential for reduced costs in terms of predictive maintenance, and other optimisations of operations.
While increase in turbidity was expected to be directly correlated with pump wear, insights from SpiralData’s data-driven approach showed that turbidity was only weakly correlated to pump performance.
SpiralData’s anomaly detection algorithm clearly identified changes in turbidity and associated issues, including changes in water quality, allowing the City of Playford to deliver better quality water to users.
Furthermore, changes in pump behaviour were expected to be irregular during pump wear, but data discovery from pump system performance revealed that key variables create clusters for separate phases, normal pump condition before wear and when a pump is worn before failure.
SpiralData was able to train an algorithm to predict with 95% accuracy the future states of a pump to identify if it needs maintenance.
Identifying pump wear early allows the City of Playford to transition to predictive maintenance, potentially saving up to 20% across its pump assets in future.