The AWA is the main industry association that I engage with. In my experience they have consistently facilitated an active and relevant dialogue on water efficiency in a way that no other industry association has. Also the diversity of membership seems to span all spheres of the water industry - I think a 'broad church' like this is the best place to thrash out our key water management ideas and issues.
The home of water professionals
We share knowledge, connect people with purpose, and inspire positive change. Our members are connected by water.
They have diverse careers across disciplines including scientists, engineers, urban designers and planners, treatment plant operators, consultants, academics, researchers, educators, communications, community consultation, policy makers, regulators, people and culture advisors, managers, data analysts, data scientists, information technology, business development, strategists, asset managers, water and catchment managers, field workers, manufacturers, trades and more.
GET INVOLVED
Awards
Be recognised at state and national levels for inspiring projects driving a sustainable water future. Open to members and students for individual or organisation achievement.
International Partnerships
Our international program connects water professionals through aid and trade, shares Australian expertise and technology, and inspires a sustainable water future.
EVENTS & NETWORKING
Ozwater'24
-
Thursday, 13 Jun 2024
ACT Water Matters Conference 2024
The ACT Water Matters Conference is the premier event on the ACT Water industry … Read more -
Thursday, 20 Jun 2024
VIC YWP Ball 2024
Get ready to dine, drink, dress up and dance at the 2024 YWP Ball with a … Read more -
Tuesday, 30 Jul 2024
North Queensland Conference 2024
The 2024 North Queensland Conference will be hosted at Hamilton Island, with … Read more -
Wednesday, 28 Aug 2024
NSW State Conference 2024
Join us on the banks of the Murray Darling, in Albury, for this years NSW State … Read more
PRINCIPAL MEMBERS
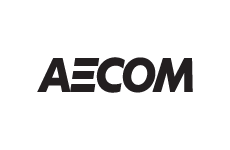
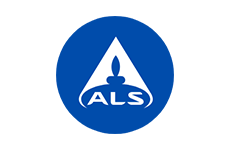
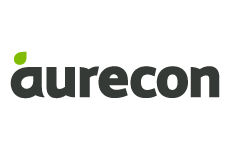

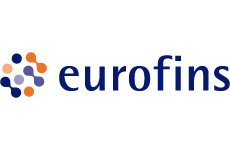
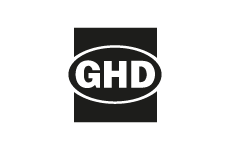
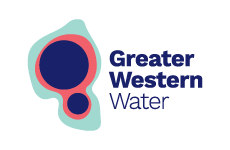
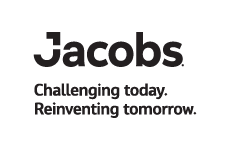
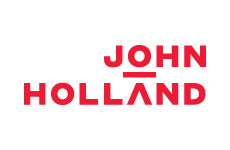
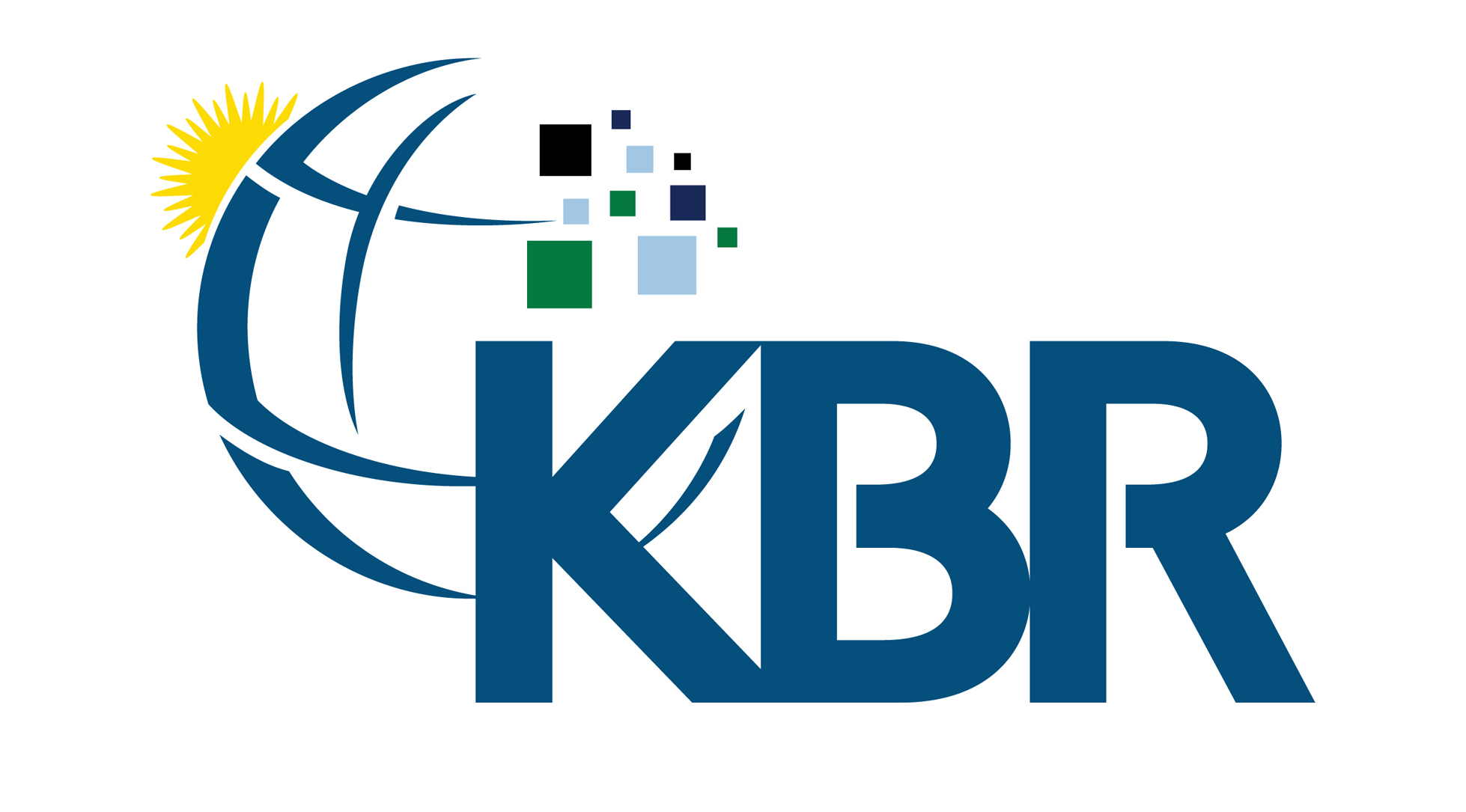
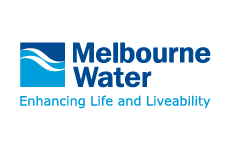
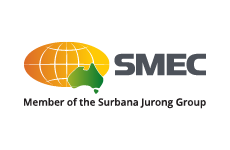
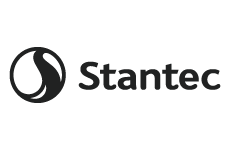
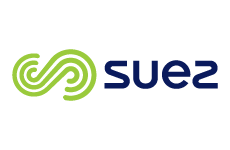
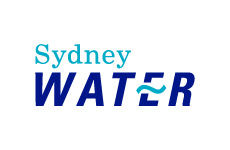
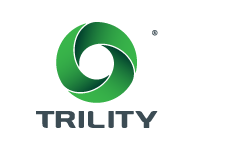
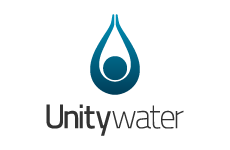
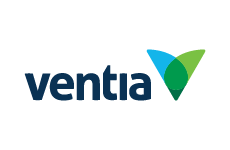
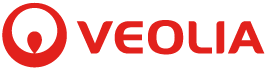
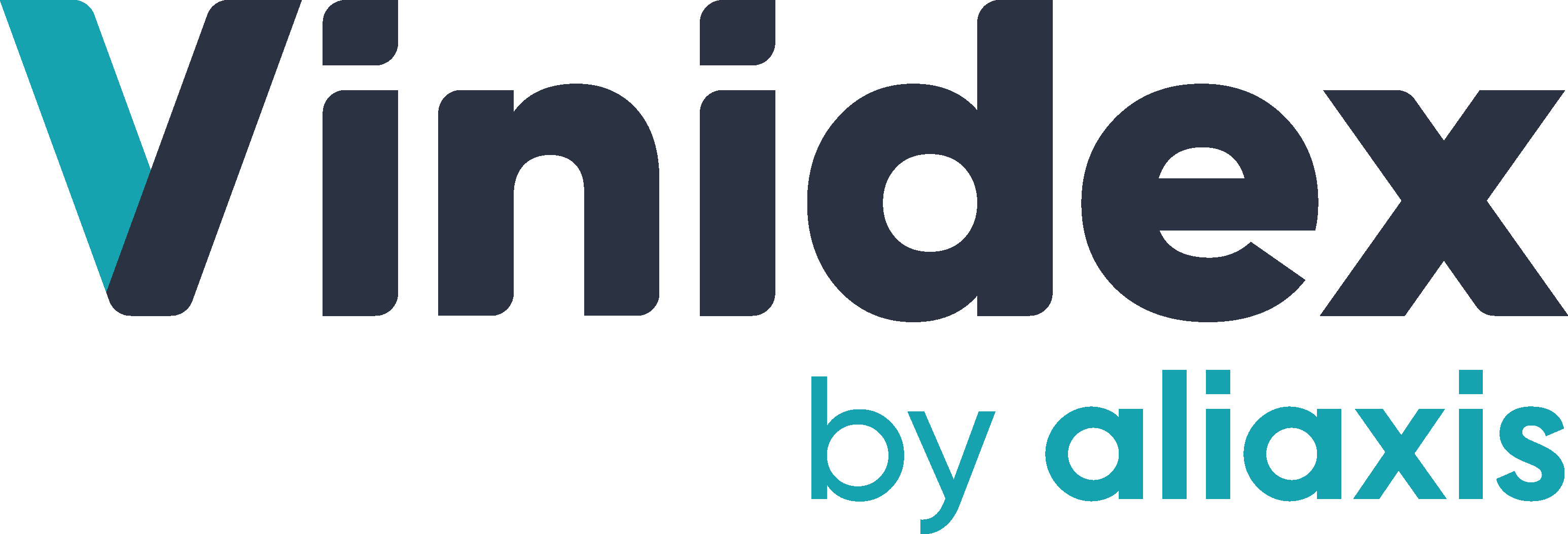
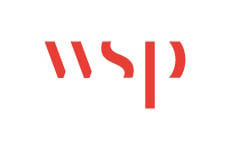
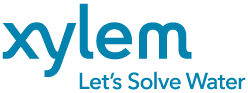
WHAT OUR MEMBERS SAY ABOUT US
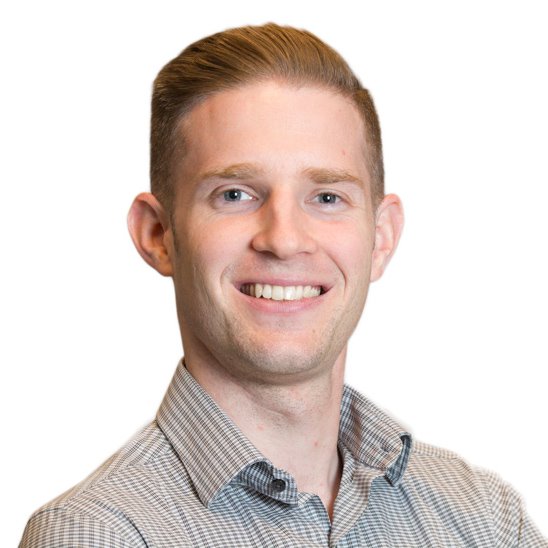
Adam Jones
Principal Engineer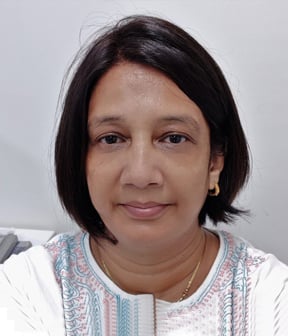
Tasneem Kanpurwala
Water Regulation OfficerAWA is a one stop shop for all water services. This includes national conferences to small networking events and webinars. Great exposure to the water world in Australia and beyond.
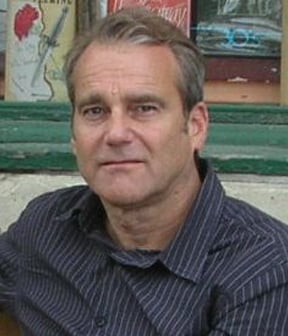
Professor Jeff Camkin
Adj. Professor (Water Resource Management)The AWA is the best forum for anyone in Australia wishing to connect with water issues as professionals or just people with an interest in water. It is the best way to meet other people interested in water issues in Australia and to support personal and professional growth in the water space.
UPDATES TO YOUR INBOX
Looking for the latest water news, insights, and events? Keen to get a better understanding of what the AWA community is? Just enter your email below to sign up.
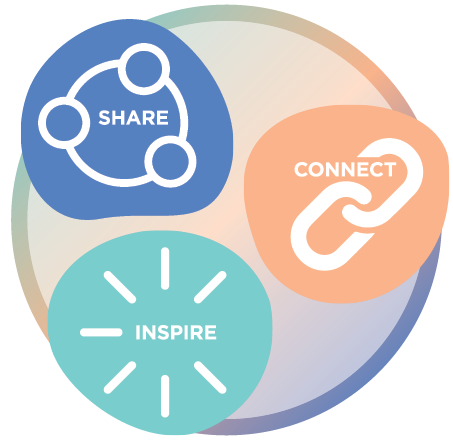